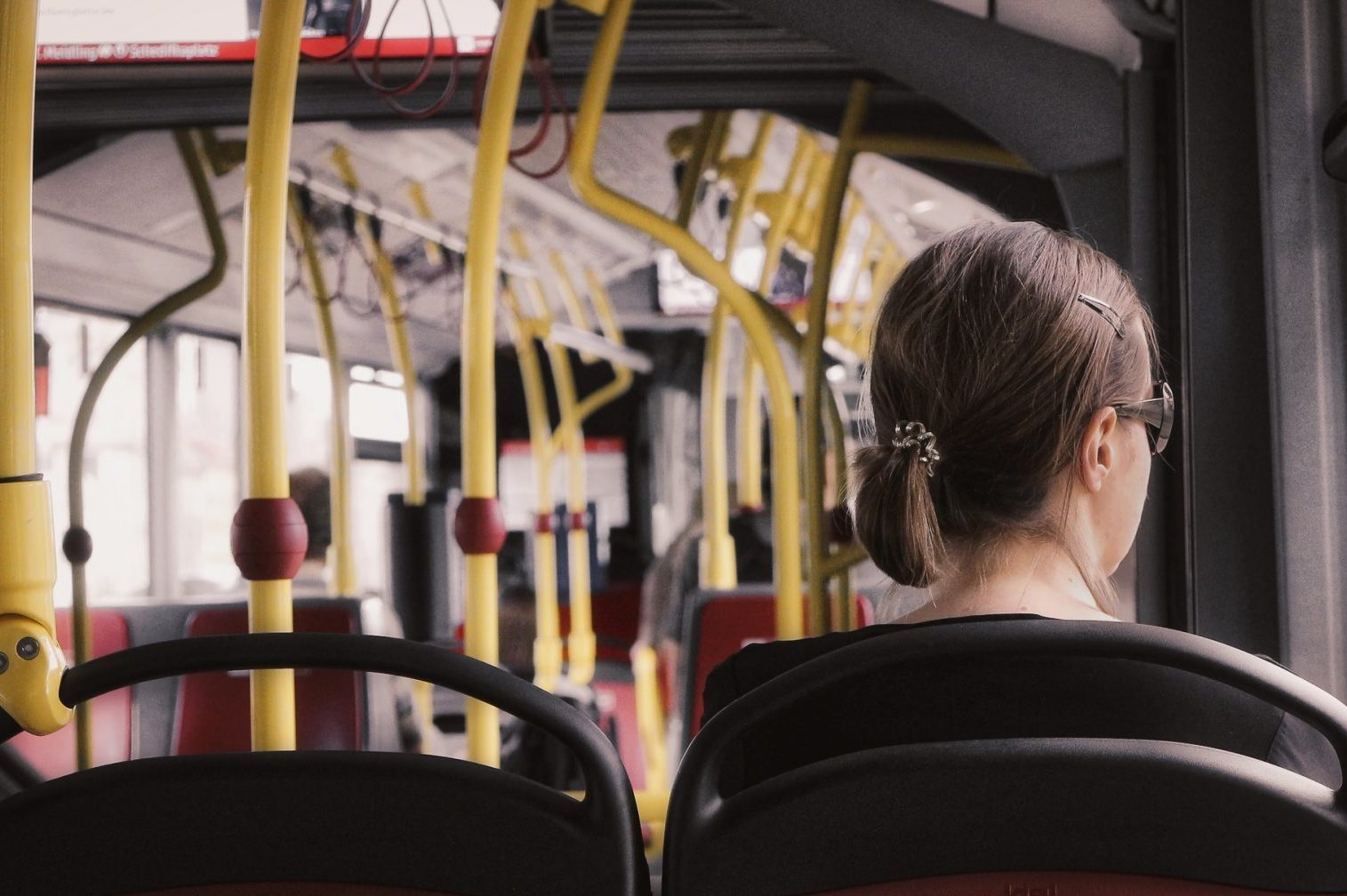
Scaling the Summit: How De-emphasizing Transit Ridership Forecasts Inadvertently Improved Ridership Forecast Accuracy
An attempt to fix one planning problem solved an altogether different one
The history of transportation planning is rife with examples of how attempts to fix one problem have created more problems somewhere else. This is a twist on that trope: a story of how a failed attempt to fix one problem became the solution to an altogether different one.
In the early 2000s, the Federal Transit Administration (FTA) experimented with a new metric to better and more clearly describe the benefits of proposed transit projects, and introduced a software package called Summit to calculate it. The new metric largely failed to more clearly convey anticipated project benefits, but it unexpectedly and substantially improved the reliability of the most straightforward measure of transit benefits: ridership. I explore this turn of events through interviews conducted in 2016 with 13 transit professionals, composed of current and past staff from six transit agencies, three consulting firms, and the FTA (or its predecessor, the Urban Mass Transportation Administration). Collectively, the interviewees had more than 300 years of experience in the transit industry.
The problem with ridership
Ridership is the simplest way to measure the public benefits of public transit. Transit systems exist to move passengers, so the more that people ride, the greater the benefit. In prioritizing possible transit infrastructure projects, perhaps the simplest approach is to rank them based on projected ridership generated relative to its cost. When the federal government first got involved in funding new rail transit systems in the 1960s, this was the approach used. However, when these early urban rail projects started opening in the 1980s, a major problem emerged: The experts making ridership predictions were not very good at it.
In 1989, Don Pickrell published a study that painted a damning picture of the state of ridership forecasting for urban rail projects. In comparing observed ridership to forecasts for all 10 federally funded rail transit projects in operation, he found that forecast ridership exceeded actual ridership by an average of 65 percent, and as much as 85 percent. The effects of these forecasting failures continue to be felt to today. As one transit professional told me: “The Pickrell report documented some really horrendous misses, which is a reputation that the program has struggled to shake in all the years that have gone by since.”
Given that so many transit infrastructure investments had apparently been made on the basis of wildly inaccurate ridership forecasts, staff at transportation agencies responsible for allocating transit project funding looked for ways to improve forecast accuracy. As one contemporary observer told me about the reaction of federal officials to consistently over-optimistic patronage forecasts:
I remember the Pickrell report back in the 1980s. … [In response], the Feds have said, “Alright, if the concern is that travel forecasting isn’t being done right, we’re going to have to spend a lot more time looking at it. …” So I think FTA has gotten more rules in as an equal and opposite reaction to other people coming in and being wide-eyed and rosy-colored glasses.
Even before the publication of the Pickrell report in 1989, Congress had authorized the Project Management Oversight program to hire independent consultants to monitor local transit agencies’ development of federally funded projects to ensure that schedules and budgets were reasonable and that transit agencies adhered to them. However, this oversight did not extend to ridership forecasts, partly because they are too complex to be easily audited by people who were not involved in the process. In some cases, when the person preparing the forecasts was not involved in developing the model used to generate them, even the forecaster might not know whether the model was appropriate to the scenario being forecast. In such cases, the forecasters treat the model as a “black box” into which they input project information and accept the output ridership forecasts without knowing much about the assumptions and processes behind them.
This modeling process is incredibly complex, with opportunity to introduce error — either intentionally or accidentally — at each stage. Ridership forecasts are commonly based on regional travel demand models. Forecasters and modelers develop and maintain these models to describe all travel within a region, and apply them to a wide variety of transportation planning decisions. Travel demand models use a series of several regression equations to estimate the total number of trips that people are predicted to take between every possible pair of origin and destination neighborhoods within the region, as well as the share of trips by each travel mode (e.g., transit, driving, walking) and the specific routes travelers are expected to take.
This modeling process is incredibly complex, with opportunity to introduce error — either intentionally or accidentally — at each stage.
At each stage of the modeling process, the forecaster must make assumptions about future changes in the population and economic characteristics of the region, and how people will respond to changes in travel times and costs. The output of one step in the modeling process is the input to the next. Thus, even small differences in assumptions (or math errors or typos) can be magnified with each step, having a large effect on the total ridership estimate.
Troublingly, forecast errors may not always be the result of technical errors. Forecasters may intentionally introduce error into forecasts in response to implicit or explicit pressure from clients or employers who wish to see proposed projects cast in a favorable light. In some cases, the distinction between mistakes and deliberate distortions might be unclear. If the ridership forecast for a project is surprisingly low, the forecaster (whose client or employer might hope the forecast will justify the project) can analyze the model to determine whether the low ridership forecast is the result of an error. However, if a ridership forecast is surprisingly high, the forecaster might just accept it as good news, rather than expending resources to look for an error. According to one forecaster I interviewed:
Despite our best efforts, sometimes there are errors. … As we’re doing these projects, even though they take years to go through the planning process, it seems like every time … we need a decision made and we’re putting together the data, things get rushed. … And it seems like every time we do a new model run, we find something that we were missing before. … They’re not just tweaks, but they’re catching omissions or errors. … Those are the types of quick things that should be done regardless of where you are, but just because of time constraints, you may not focus on them unless you’re running into issues with [low ridership].
Transit planners may lack motivation or resources to rigorously detect and correct for modeling errors, and these potentially flawed models are often reused for many projects over time. Given these factors, Pickrell’s finding that ridership forecasts were often wildly inaccurate comes as no surprise.
Looking beyond ridership
In reaction to the demonstrated failures of ridership forecasts and subsequent attempts to improve their accuracy, some practitioners argued that emphasizing ridership as the sole or central measure of a project’s potential benefits might be misplaced. Couldn’t new transit projects generate benefits beyond just attracting new riders? Certainly. Transit projects might contribute to economic development or congestion relief, for example. But the economic development benefits of transit flow largely from people riding transit — to work or shop at local businesses. Congestion relief is likewise achieved when travelers choose to ride transit rather than drive their own vehicles.
But not all transit project benefits flow from added riders. Transit projects can improve service for existing riders, which isn’t measured by the number of new riders. In 2001, the FTA introduced Transportation System User Benefits (TSUB) to replace ridership as the primary measure of benefits in proposed transit projects. This new measure combined the projected travel time savings for existing riders with the number of new riders to produce a dollar value of the total project benefits. Although the logic behind this calculation had a firm theoretical basis in microeconomics, it was less intuitive for those without an economics background. Relative to a simple ridership metric, the TSUB metric was hard to understand or succinctly explain.
One transit manager described how the ranking of transit projects changed when travel time savings were incorporated into the measure of project benefits:
When I started working in project development, it was a pretty simple calculation of cost versus ridership. … Then FTA changed that to look at user benefits. And user benefit measured whether there were travel-time savings that happen from the project. When they went from just riders to that travel-time saving measure, it really changed the kinds of projects that could qualify for New Starts funds. It really benefitted long-haul light-rail projects. It benefitted commuter rail projects. Streetcar projects didn’t really show particularly well because they’re not really saving anybody travel time.
On the other hand, a consultant observed that, perhaps surprisingly, projects performing well by one measure generally performed well by the other:
We did a little exercise to see how cost per hour of user benefit … correlated with cost per project trip, just to see if it really changed the playing field. To my surprise, it really didn’t. The ones that were good under the old measure are still good under the new measure. So maybe it’s okay.
If selecting projects based on the projected number of new riders produces roughly the same outcome as a complicated user benefits measure, then why bother with the more complicated measure? Ultimately, the complexity of the TSUB metric was its undoing. One transit manager I interviewed described how he never really understood what the TSUB measure was supposed to represent, even after sitting down with economists to have them explain it to him. Another explained how the concepts behind the TSUB measure were so complicated that when Congress passed the Moving Ahead for Progress in the 21st Century Act (MAP-21) — its surface transportation bill in 2012 — they required FTA to abandon TSUB in favor of a return to a simpler measure of ridership.
I think the switch to user benefits was significant because it did try to capture all of the transportation benefits of a project, not just new riders. But in part it was the seeds of its own undoing because it got really complicated and it required sophisticated modeling. … In MAP-21, Congress said “Enough of that! That’s too complicated!” I think this administration was also trying to step away from that kind of a measure. We ended up with cost per project trip, which to me is a step backwards because I don’t think that measure is a particularly good indicator of benefit at all. You can have a lot of people riding on a project, but are they better off?
In the end, TSUB may have been a more complete measure of project benefits than ridership alone, but it was too complicated to convey those benefits in a meaningful way. On the other hand, it was complicated enough to address a major fault of using ridership as a measure of future project benefits: a lack of confidence in ridership forecasts.
Summit saves the day
The TSUB metric wasn’t just complicated to understand — it was complicated to calculate, especially for a forecaster who had become accustomed to treating a travel demand model as a “black box.” To make things easier, the FTA introduced a software package called Summit in 2003 to assist project sponsors in calculating the TSUB metric.
An FTA staff member described how the introduction of the Summit software package was a watershed in evaluating the underlying assumptions used in ridership forecasts. Although the purpose of Summit was to assist project sponsors in computing travel time savings, it had the additional effect of providing greater transparency about a travel demand model’s underlying assumptions:
An ancillary, but it turned out — in my view anyway — a more important result of the Summit software was that, for the very first time, it produced detailed reporting of the ridership forecasts. That was the equivalent of shining a light into a really dark box, and there was all sorts of pretty ugly stuff going on that you would normally have a very hard time finding because of the complex nature of ridership forecasting. … There were all sorts of unintended things happening. And all of a sudden, the ridership stuff got a lot more rigorous.
A consultant confirmed that, although Summit was no longer used after the requirement to report travel time savings was discontinued by FTA, it had a permanent effect on the accuracy of ridership forecasts. Summit allowed modelers to correct persistent errors in their models and improve their knowledge and understanding of travel-demand modeling:
[Summit] was a big game-changer because you could actually identify and describe the major problems that the model had. Previously, you would just be lost and swimming in too many numbers, and you couldn’t actually figure out what the hell was going on except at a very deep level. Summit allowed you to look at it … and actually see that the model’s not doing a very good job at all. It found that the model-development practice and model-application practice was pretty bad. And I would say bad to the point of being almost criminal or fraudulent. … That was a complete watershed moment. We unlearned more about what we knew than I had ever learned. I’ve learned more about forecasting in the last 13 years than I had in the previous 13, by a country mile.
Summit allowed modelers to correct persistent errors in their models and improve their knowledge and understanding of travel-demand modeling.
Improving existing travel demand models (and forecasters’ understanding of them) was not the intended purpose of Summit. However, many persistent modeling errors that had been difficult to catch in black box models became obvious — and easier to correct — when forecasters started using Summit. The software thus became a much-needed source of quality control for travel demand models. This unintended benefit of Summit was soon recognized by federal staff evaluating proposed transit projects. In testimony before Congress in 2004, the inspector general of the Department of Transportation described the Summit software as “an important step … to help identify problems with ridership forecasts.”
The switch back to ridership
With the passage of a new federal surface transportation bill (MAP-21) in 2012, Congress abandoned the TSUB as a measure of project benefits, opting instead for returning to ridership as a simpler, albeit less comprehensive, performance measure. However, the decade of experimentation with TSUB forced forecasters to examine their models and ultimately improve the reliability of ridership forecasts.
For the 15 federally funded new rail projects completed between 2008 and 2011, forecasts still exceeded observed ridership by an average of 48 percent, but this was an improvement over the average error of 65 percent that Pickrell had found for projects in the 1980s. An even more promising sign is that, where Pickrell had found that ridership forecasts were higher than actual ridership in every case, four of the 15 projects (27 percent) that opened between 2008 and 2011 had actual ridership that was higher than the forecasts.
Furthermore, a 2016 paper by David Schmitt found that there was a significant improvement in forecast accuracy for transit projects that opened after 2007 — the year in which projects incorporating forecasts completed after the introduction of Summit first began to open for service. In trying (and perhaps failing) to come up with a measure of project benefits to replace ridership, the FTA improved the usefulness of ridership forecasts as performance measures.